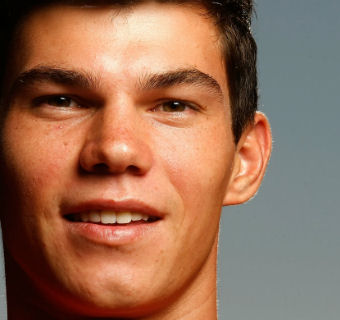
Consistency is a big part of AFL Dreamteam. All of us know the pain of winning an eliminator by 10 points because player A gets 140, then losing by 30 when he gets 70 next round. That’s why when you pick your teams; consistency and ceiling combined have to come into it. The team that wins the competition at the end of the year is the team that, week after week, pumps out 2200+.
In the first edition of my consistency series, I looked at most of the popular premiums in each position, analysed and then ranked them according to their consistency. This week it is the rookie’s turn. One of the most prevalent questions on the @DTTalk website often reads something like this: “Should I play Mayes or Staker this week?” or “Vlastuin or O’Meara?”. Especially in the lockout post. 90% of the questions in there relate to that.
And I thought to myself after the previous edition, ‘why not address that, much like I addressed the premium dilemma’. And that is why you are now reading the second edition of Consistency. I have compiled about 25 odd rookies, the most popular in each position, and then calculated the stats for each category I rank them in. The result is, like last time, 4 tables. However, I have included two more categories into the table this time, based on the suggestions from the comments last week. They are the Weak Mean and the Coefficient of Variation. They are accompanied by the mean, affected mean, standard deviation, range and IQR. As usual, I will explain them here:
- The first stat is the Normal Meanor just the mean. It is the sum of every single score the player has got divided by the amount of times he has played.
- The second category is the affected mean. This takes into the account only the scores from the games where the player has played the full game i.e. not been subbed or injured. A game score is still included here if the player was just bad (step up Scotland buddy). However, the games where the opposition team is Gold Coast, Melbourne or Greater Western Sydney is not included. That is the next category. Every other category apart from the weak mean and normal mean is based on the values from this category, i.e. the range is not going to include sub-affected scores.
- The third group is the Weak Mean. It is average score of the games played against GWS, GC or Melbourne. This group is included to highlight the difference between scoring from the weak teams and strong-er teams to better help you predict the scoring output.
- The fourth stat is the standard deviation. It is a measure of how far the data is spread out. Lower values are better, i.e. the lower the value, the more consistent the player. This is the set of values I rank the players on.
- Next is the range. The difference between the highest and lowest scores, i.e. Max take away Min. Lower is better.
- The sixth measure of data is the Interquartile Range, or IQR. This value is given to you in the form A-B (the dash does not mean minus, it means ‘in between’, i.e. between A and B). This gives you the range the player scores 50% of his scores in. For example, say Player D has an IQR of 100-130. This means that player D scores between 100 and 130 fifty percent of the time he plays. The lower the number between the values I give you, the better. This means you want the values to be as close together as possible.
- The final measure of data is called the Coefficient of Variation, or just COV. I highly recommend you do not read the explanation on Wikipedia on this because they overcomplicate it to an extreme level. It is basically the ratio of the standard deviation to the affected mean (by that I mean SD divided by the AM). This value is used to correlate the mean and SD. For example, in my last article, I wrote that Hibberd had a lower standard deviation then Goddard. However, Goddard has a higher mean. This, according to the reader, may affect their choice of picking a player i.e. is it ok to pick a player with a higher SD if he also has a higher mean? Well this value kind of equates them to the same level. Hibberd has a COV of 0.16. Goddard has a COV of 0.15. Therefore, Goddard’s higher mean compensates for the fact that he also has a higher SD. Massive thanks to the commenter who introduced this to me, much appreciated.
General Info
Okay! Now we have got that sorted out, we can move into the tables! Remember, they are ranked by standard deviation not average, and the green numbers on the left side are their places if you were placing them by average. They are there to contrast between the highest averaging players and the players with the lowest standard deviation. Lastly, I will comment on a couple of players of interest in each category.
Defenders
An interesting player to note here is Webster, who has played 2 games that are eligible for the affected mean. This means his data may be slightly inaccurate as we haven’t been able to see a massive range of scores. This is highlighted by the fact that there is daylight between his SD and the next person on the list, Vlastuin. In fact, I would suggest his SD is an outlier, but I still included it in the table for comparison. You can see that everyone else’s SD is around the 17-18 mark. This is about what you should expect.
I would recommend either playing either Vlastuin or Goodes out of these 6, because everyone else has either a high SD or a high COV. This could mean they have low SDs, but also have very low affected means. I would not recommend playing anyone else except for Terlich, unless necessity dictates that you should.
Disclaimer: Do not come knocking down my door like you did to Calvin the other day because you don’t play Terlich and then he bangs out a hundred. My recommendations are based purely on stats. I cannot predict the future, neither I’d wager, could anyone else frequenting this page.
Ruckmen
Yeah look, I’m not going to sugar coat this: there is nothing to write home about here. In fact, I highly recommend you do not play Blicavs or Daw (especially Daw!!!!! There is a reason the Kangaroos are being dubbed the South African Cricket team. Actually chuck Stosur in there as well. Chokers, the lot of them) unless the bye rounds dictate you must do so. Wilbur (in the words of @MattCraigDT) Minson and Kreuzer both got scores in excess of 110 on the weekend, I would have a look at one of those two at least, they are my two ruckmen.
Forwards
Okay, let’s move forward. The forwards are quite interesting. The player with the highest affected mean is S. Dwyer, but he is ranked last for consistency. Based on his last few scores, it is easy to see why. 95, 10something and then 65. Stay away.
Staker is holding the fort at number one, however, be aware that he has played a total of 3 games. Don’t judge him yet. You must all be thinking, “How did Mayes get in at number 2?” Let me explain. Look at his weak mean. 103. Now look at his affected mean. 64.67. Put simply, he is a bigger flat-track bully then the Indian Cricket team (barring perhaps Dhoni, Kohli and Karthik). He slays the weaker teams, but doesn’t do so well against stronger teams. His weak team scores aren’t included in the affected mean and that is why he has a low standard deviation. However, based on form, my number one pick has to be Macaffer. (I’m going against the stats *collective gasp*) He’s flourishing, and has a great IQR of between 60 and 79. Get on board and play him.
Midfielders
Okay, before we go any further I would like to explain what some of you are already dubbing an ‘anomaly’ at the top of the list. Mr M. Hutchings. He has played 3 games, two of which are valid for the affected mean. In those two games he scored 83 and 84. If he keeps this up, he is a massive beast and jump on. However, judging from the amount of tweets on Monday night that contained his name and an expletive in the same sentence, I would imagine he wouldn’t be around much longer.
Viney probably isn’t even playing, but if he comes back, look at his stats. O’Meara. The bald magician of the future. It is simple. If you’ve got him, play him. If you don’t I’m not sure why you are even reading this post. The kid is a jet, not the heavy, awkward Boeing 747; he is the epitome of a fast, nimble Blackbird. He is rapidly ascending so watch your feet Mr Hartlett.
Wines isn’t the beast he once was, his scores have been rapidly decreasing. Seedsman has snuck under a lot of people’s radars. He has gunned it in the last few weeks, and watching the last few games (my brother goes for Collingwood, so I have a polite interest) I’m surprised he doesn’t have more; he is involved in almost everything they do. I think it is fairly safe to say he will get you at least an 80 most weeks.
Conclusion
Whew! Okay, now that’s done, I can show you how you would use these tables when looking when to play someone on the field. If you are looking for higher averages when playing players, then ignore this article completely. However, if you are smarter and want consistency over flashy scores now and then, you would do well to study these tables.
For example:“Hey guys, just wondering which of these two players would u play, Paparone or Neade?” Okay, first of all it’s ‘you’, not ‘u’, is it really that hard to write two extra letters? Second, I would check the teams of Thursday, more than likely one of those two would be out. Thirdly, if both of them are playing, I would definitely be going Neade, he has a higher mean and lower SD.
Okay, so now you know how to use this, you can go forth and use it. Remember, just because I haven’t included a player in this table, doesn’t mean you don’t want to play them, it just means they weren’t included in my list because I just picked the ones that mostly everyone has.
Cheers, and any questions, just hit me up on Twitter@ZeusODeaDT or comment and I will try my best to answer them.
Cheers,
Zeus
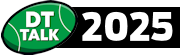
Recent Comments